AI
Leveraging Machine Learning and Artificial Intelligence for 5G
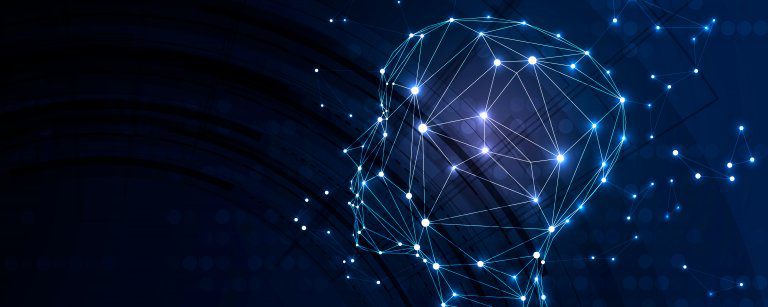
The heterogenous nature of future wireless networks comprising of multiple access networks, frequency bands and cells - all with overlapping coverage areas - presents wireless operators with network planning and deployment challenges. Machine Learning (ML) and Artificial Intelligence (AI) can assist wireless operators to overcome these challenges by analyzing the geographic information, engineering parameters and historic data to:
- Forecast the peak traffic, resource utilization and application types
- Optimize and fine tune network parameters for capacity expansion
- Eliminate coverage holes by measuring the interference and using the inter-site distance information
5G can be a key enabler to drive the ML and AI integration into the network edge. The figure below shows how 5G enables simultaneous connections to multiple IoT devices generating massive amounts of data. The integration of ML and AI with 5G multi-access edge computing (MEC) enables wireless operators to offer:
- High level of automation from the distributed ML and AI architecture at the network edge
- Application-based traffic steering and aggregation across heterogeneous access networks
- Dynamic network slicing to address varied use cases with different QoS requirements
- ML/AI-as-a-service offering for end users
ML and AI for Beamforming
5G, deployed using mm-wave, has beam-based cell coverage unlike 4G which has sector-based coverage. A machine learned algorithm can assist the 5G cell site to compute a set of candidate beams, originating either from the serving or its neighboring cell site. An ideal set is the set that contains fewer beams and has a high probability of containing the best beam. The best beam is the beam with highest signal strength a.k.a. RSRP. The more activated beams present, the higher the probability of finding the best beam; although the higher number of activated beams increases the system resource consumption.
The user equipment (UE) measures and reports all the candidate beams to the serving cell site, which will then decide if the UE needs to be handed over to a neighboring cell site and to which candidate beam. The UE reports the Beam State Information (BSI) based on measurements of Beam Reference Signal (BRS) comprising of parameters such as Beam Index (BI) and Beam Reference Signal Received Power (BRSRP). Finding the best beam by using BRSRP can lead to multi-target regression (MRT) problem while finding the best beam by using BI can lead to multi-class classification (MCC) problem.
ML and AI can assist in finding the best beam by considering the instantaneous values updated at each UE measurement of the parameters mentioned below:
- Beam Index (BI)
- Beam Reference Signal Received Power (BRSRP)
- Distance (of UE to serving cell site),
- Position (GPS location of UE)
- Speed (UE mobility)
- Channel quality indicator (CQI)
- Historic values based on past events and measurements including previous serving beam information, time spent on each serving beam, and distance trends
Once the UE identifies the best beam, it can start the random-access procedure to connect to the beam using timing and angular information. After the UE connects to the beam, data session begins on the UE-specific (dedicated) beam.
ML and AI for Massive MIMO
Massive MIMO is a key 5G technology. Massive simply refers to the large number of antennas (32 or more logical antenna ports) in the base station antenna array. Massive MIMO enhances user experience by significantly increasing throughput, network capacity and coverage while reducing interference by:
- Serving multiple spatially separated users with an antenna array in the same time and frequency resource
- Serving specific users with beam forming steering a narrow beam with high gain to send the radio signals and information directly to the device instead of broadcasting across the entire cell, reducing radio interference across the cell.
The weights for antenna elements for a massive MIMO 5G cell site are critical for maximizing the beamforming effect. ML and AI can be used to:
- Identify dynamic change and forecast the user distribution by analyzing historical data
- Dynamically optimize the weights of antenna elements using the historical data
- Perform adaptive optimization of weights for specific use cases with unique user-distribution
- Improve the coverage in a multi-cell scenario considering the inter-site interference between multiple 5G massive MIMO cell sites
ML and AI for Network Slicing
In the current one-size-fits-all approach implementation for wireless networks, most resources are underutilized and not optimized for high-bandwidth and low-latency scenarios. Fixed resource assignment for diverse applications with differential requirements may not be an efficient approach for using available network resources. Network slicing creates multiple dedicated virtual networks using a common physical infrastructure, where each network slice can be independently managed and orchestrated.
Embedding ML algorithms and AI into 5G networks can enhance automation and adaptability, enabling efficient orchestration and dynamic provisioning of the network slice. ML and AI can collect real time information for multidimensional analysis and construct a panoramic data map of each network slice based on:
- User subscription,
- Quality of service (QoS),
- Network performance,
- Events and logs
Different aspects where ML and AI can be leveraged include:
- Predicting and forecasting the network resources can enable wireless operators to anticipate network outages, equipment failures and performance degradation
- Cognitive scaling to assist wireless operators to dynamically modify network resources for capacity requirements based on the predictive analysis and forecasted results
- Predicting UE mobility in 5G networks allowing Access and Mobility Management Function (AMF) to update mobility patterns based on user subscription, historical statistics and instantaneous radio conditions for optimization and seamless transition to ensure better quality of service.
- Enhancing the security in 5G networks preventing attacks and frauds by recognizing user patterns and tagging certain events to prevent similar attacks in future.
With future heterogenous wireless networks implemented with varied technologies addressing different use cases providing connectivity to millions of users simultaneously requiring customization per slice and per service, involving large amounts of KPIs to maintain, ML and AI will be an essential and required methodology to be adopted by wireless operators in near future.
Deploying ML and AI into Wireless Networks
Wireless operators can deploy AI in three ways:
- Embedding ML and AI algorithms within individual edge devices for to low computational capability and quick decision-making
- Lightweight ML and AI engines at the network edge to perform multi-access edge computing (MEC) for real-time computation and dynamic decision making suitable for low-latency IoT services addressing varied use case scenarios
- ML and AI platform built within the system orchestrator for centralized deployment to perform heavy computation and storage for historical analysis and projections
Benefits of Leveraging ML and AI in 5G
The application of ML and AI in wireless is still at its infancy and will gradually mature in the coming years for creating smarter wireless networks. The network topology, design and propagation models along with user’s mobility and usage patterns in 5G will be complex. ML and AI can will play a key role in assisting wireless operators to deploy, operate and manage the 5G networks with proliferation of IoT devices. ML and AI will build more intelligence in 5G systems and allow for a shift from managing networks to managing services. ML and AI can be used to address several use cases to help wireless operators transition from a human management model to self-driven automatic management transforming the network operations and maintenance processes.
There are high synergies between ML, AI and 5G. All of them address low latency use cases where the sensing and processing of data is time sensitive. These use cases include self-driving autonomous vehicles, time-critical industry automation and remote healthcare. 5G offers ultra-reliable low latency which is 10 times faster than 4G. However, to achieve even lower latencies, to enable event-driven analysis, real-time processing and decision making, there is a need for a paradigm shift from the current centralized and virtualized cloud-based AI towards a distributed AI architecture where the decision-making intelligence is closer to the edge of 5G networks.
The Role of CableLabs
The cable network carries a significant share of wireless data today and is well positioned to lay an ideal foundation to enable 5G with continued advancement of broadband technology. Next-generation wireless networks will utilize higher frequency spectrum bands that potentially offer greater bandwidth and improved network capacity, however, face challenges with reduced propagation range. The 5G mm-wave small cells require deep dense fiber networks and the cable industry is ideally placed to backhaul these small cells because of its already laid out fiber infrastructure which penetrates deep into the access network close to the end-user premises. The short-range and high-capacity physical properties of 5G have high synergies with fixed wireless networks.
A multi-faceted CableLabs team is addressing the key technologies for 5G deployments that can benefit the cable industry. We are a leading contributor to European Telecommunication Standards Institute NFV Industry Specification Group (ETSI NFV ISG). Our SNAPS™ program is part of Open Platform for NFV (OPNFV). We are working to optimize Wi-Fi technologies and networks in collaboration with our members and the broader ecosystem. We are driving enhancements and are standardizing features across the industry that will make the Wi-Fi experience seamless and consistent. We are driving active contributions to 3GPP Release 16 work items for member use cases and requirements.
Our 10G platform complements 5G and is also a key enabler to provide the supporting infrastructure for 5G to achieve its full potential. CableLabs is leading the efforts for spectrum sharing to enable coexistence between Wi-Fi and cellular technologies, that will enable multi-access sharing with 3.5 GHz to make the 5G vision a reality.