AI
A Different Future for Artificial Intelligence
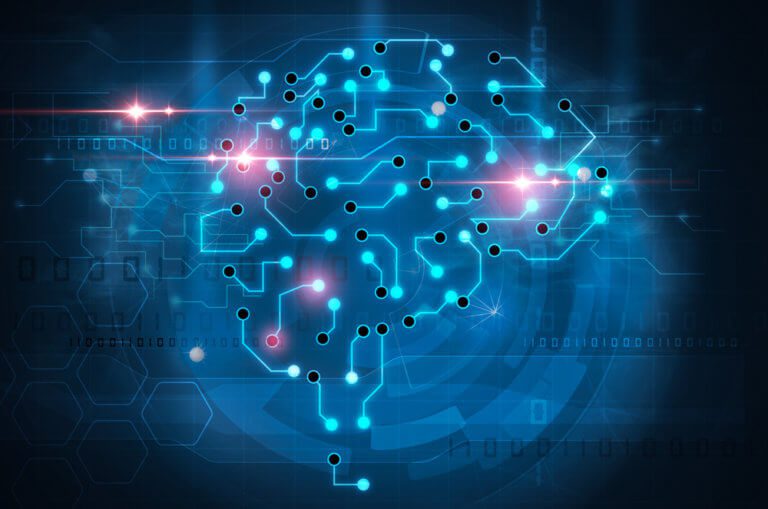
Not a single day goes by without us hearing about AI. Machine learning, and AI, as these terms are often conflated, have become part of the lexicon in business, technology and finance. Great strides in pattern recognition and the discovery of hidden correlations in vast seas of data are fueling the enthusiasm and hopes of both the technical and business communities.
While this success is worth celebrating, we should not lose track of the fact that there are many other aspects of artificial intelligence beyond machine learning. Common sense reasoning, knowledge representation, inference, to name a few, are not part of the toolbox today but will have to be if we seek forms of machine intelligence that have much in common with our own.
The reason why such forms of machine intelligence are not being used is due to the difficulty that those problems entail. Unlike the recent advances in machine learning, half a century of research in symbolic systems, cognitive psychology and machine reasoning have not produced major breakthroughs.
Intelligent Networks: A New Form of Artificial Intelligence
A more promising future can be expected once we realize that intelligence is not restricted to single brains; it also appears in groups, such as insect colonies, organizations and markets in human societies, to name a few. In all these cases, large numbers of agents capable of performing local tasks that can be conceived as computations, engage in collective behavior which successfully solves a number of problems that transcend the capacity of a single individual to solve. And they often do so without global controls, while exchanging information that is imperfect and at times delayed.
Many of the features underlying distributed intelligence can be found in the computing networks that link our planet. Within these systems processes are created or “born”, migrate across networks and spawn other processes in remote computers. And as they do, they solve complex problems -think of what it takes to render a movie in your screen -while competing for resources such as bandwidth or CPU contested by other processes.
Interestingly, we understand the performance of distributed intelligence, both natural and artificial, much better than the workings of individual minds. This is partly due to the ease with which we can observe and measure the interactions among individuals and programs as they navigate complex informational spaces. Contrast this with the difficulty in learning about detailed cognitive processes within the human brain. And from this large body of knowledge we know that while the overall performance of a distributed system is determined by the capacity of many agents exchanging partial results that are not always optimal, success is determined by those few making the most progress per unit time (think of many agents looking for the proverbial needle in the stack).
Distributed Intelligence: Better than the Best
This suggests a promising path forward for AI; the creation of distributed intelligent programs that can sense, learn, recognize and aggregate information when deployed throughout the network in the service of particular goals. Examples can be the sensing of local anomalies that are aggregated intelligently in order to decide on a given action, the collective detection of malware in parts of the network, sensor fusion, and effective responses to predetermined traffic and content patterns, to name a few.
Distributed AI is not an illusory goal. A few such systems have already been designed and tested and have shown large improvements in the times needed to solve hard computational problems such as cryptarithmetic and graph coloring. These are problems characterized by the fact that as their size increases linearly, the time to finding a solution rises exponentially. A common example is the traveling salesman problem, which can be seen as a metaphor for the laying of networks in such a way that they minimize the number of traversals needed to cover a number of cities and users. While there are a number of powerful heuristics to approach this optimization problem, for large instances one can only hope for solutions that while not optimal, satisfy a certain number of constraints.
The beauty of cooperative systems is that once deployed, they can exhibit combinatorial implosions. These implosions are characterized by a sudden collapse in the number of possible venues to a solution due to the effectiveness of cooperation, and as a result, what took exponential times to solve is now rendered in linear or polynomial time. These implosions appear as both the quality and the number of messages exchanged by AI agents increases while working on the solution of complex problems.
In closing, the emergence of distributed AI will allow for the solution of a number of practically intractable problems, many of them connected with the smooth and safe functioning of our cable networks. Imagine applying different AI solutions to search for security anomalies and combining them in order to identify and act on them. Or monitoring distal parts of the network with different kind of sensors whose outputs are aggregated by intelligent agents. These are just two instances of the myriad problems that could be tackled by a distributed form of artificial intelligence. The more examples we think of and implement, the closer we will get to this vision of a society of intelligent agents who, like the social systems we know, will vastly outperform the single machine learning algorithms we are so familiar with.
Subscribe to our blog to learn more about the future of artificial intelligence.